Main content
Home
Menu
Background: In early 2020 a global pandemic of COVID-19, a severe respiratory illness caused by infection with the SARS-CoV-2 virus, was declared by the World Health Organization. As an emergent pathogen, little is known about how best to manage it, and as of March 21st 2020 there were no known effective treatments. It is apparent that there is an urgent need to determine what works, what doesn't, and in whom, and that piecemeal approaches to evidence accumulation and knowledge dissemination through peer-reviewed publication, even if expedited, will be inadequate for mitigating the impact of the pandemic. In response, we are working toward a learning health systems approach to the broad and rapid accumulation of data, its conversion to knowledge, and in turn to improvements in healthcare. This work is intended to compliment evidence generated through randomised clinical trials, with existing expert knowledge, and real-world data.
We are: a collaborative group of clinicians, mathematical modelers, computer scientists, epidemiologists, public health researchers and trialists aiming to improve the clinical management of COVID-19. The site is curated by Adaptive Health Intelligence. To request write priviledges, please contact jessica.ramsay@telethonkids.org.au. Non-contributors can request access from Jess by emailing her with a health (.health) or academic (.edu) email address.
Research aim: To improve the management of COVID-19 using Bayesian inference to rapidly learn from accumulating clinical data.
Disclaimer: This work is created in good faith. It is intended to be used to guide trial design, and decision-making by experienced clinicians, supplementing rather than replacing their clinical judgement, expert guidelines and local recommendations. In light of the speed and impact of the pandemic, we develop this within the Open Science Framework in order to invite and encourage rapid, transparent critical feedback and to expedite sharing of the outputs, in place of traditional peer-review. The contributers cannot bear responsibility for treatment decisions made.
Short-term outcomes: Better understanding of COVID-19 and a consensus on the critical clinical uncertainties that need to be resolved to mitigate the impact of the pandemic, thereby allowing for optimal focus of the research effort.
Longer-term outcomes: Better individualised management of patients with suspected or proven COVID-19 infection .
Site layout: This site is divided into sections, including a section covering the development of Bayesian networks to capture accumulating knowledge for decision support, and sections reflecting each of the different contexts of management.
- Community-based management of typical infection
- Hospital-based management of severe but non-critical infection
- ICU-based mangement of extremely sever infection
- Post-exposure prophylaxis of close contacts
- Prophylaxis of healthcare workers
Data: It is not intended that this OSF site will serve as a repository for raw, line-listed clinical data. The BN tool will be designed to accommodate data variables collected using the standard ISARIC case report form which can be downloaded from the ISARIC website.
Page permissions have changed
Your browser should refresh shortly…
Renaming wiki...
Wiki page deleted
Press Confirm to return to the project wiki home page.
Connected to the collaborative wiki
This page is currently connected to the collaborative wiki. All edits made will be visible to contributors with write permission in real time. Changes will be stored but not published until you click the "Save" button.
Connecting to the collaborative wiki
This page is currently attempting to connect to the collaborative wiki. You may continue to make edits. Changes will not be saved until you press the "Save" button.
Collaborative wiki is unavailable
The collaborative wiki is currently unavailable. You may continue to make edits. Changes will not be saved until you press the "Save" button.
Browser unsupported
Your browser does not support collaborative editing. You may continue to make edits. Changes will not be saved until you press the "Save" button.
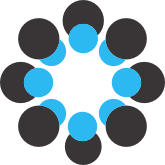
Start managing your projects on the OSF today.
Free and easy to use, the Open Science Framework supports the entire research lifecycle: planning, execution, reporting, archiving, and discovery.
Copyright © 2011-2025
Center for Open Science
|
Terms of Use
|
Privacy Policy
|
Status
|
API
TOP Guidelines
|
Reproducibility Project: Psychology
|
Reproducibility Project: Cancer Biology