Main content
Home
Menu
Loading wiki pages...
Page permissions have changed
Your browser should refresh shortly…
Renaming wiki...
Wiki page deleted
Press Confirm to return to the project wiki home page.
Connected to the collaborative wiki
This page is currently connected to the collaborative wiki. All edits made will be visible to contributors with write permission in real time. Changes will be stored but not published until you click the "Save" button.
Connecting to the collaborative wiki
This page is currently attempting to connect to the collaborative wiki. You may continue to make edits. Changes will not be saved until you press the "Save" button.
Collaborative wiki is unavailable
The collaborative wiki is currently unavailable. You may continue to make edits. Changes will not be saved until you press the "Save" button.
Browser unsupported
Your browser does not support collaborative editing. You may continue to make edits. Changes will not be saved until you press the "Save" button.
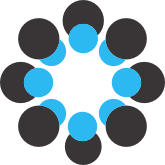
Start managing your projects on the OSF today.
Free and easy to use, the Open Science Framework supports the entire research lifecycle: planning, execution, reporting, archiving, and discovery.
Copyright © 2011-2025
Center for Open Science
|
Terms of Use
|
Privacy Policy
|
Status
|
API
TOP Guidelines
|
Reproducibility Project: Psychology
|
Reproducibility Project: Cancer Biology