Main content
Home
Menu
Zoom link: https://harvard.zoom.us/j/812428264
Here we investigate the relationship between contemporary neural models’ inductive bias, perplexity, and ability to predict human reading times. We train three model architectures---5-grams, LSTMs, and Recurrent Neural Network Grammars (which have explicit syntactic representations; Dyer et al., 2016)---on four datasets derived from the BLLIP corpus (Charniak et. al, 2000). For each model we investigate the relationship between word surprisal and human reading time on three online processing datasets: the Dundee eye-tracking corpus (Kennedy et al. 2003), self-paced reading from selections of the Brown corpus (Smith & Levy, 2013), and the Natural Stories corpus (Futrell, 2017), using multiple regression with standard control predictors (Goodkind & Bicknell, 2018). In line with previous findings, we find a strong linear relationship between surprisal and reading times for all models. Comparing across neural models trained on the same dataset, we find a negative relationship between test perplexity and predictive power. However, we find that n-gram models demonstrate predictive power comparable with the neural models despite much worse test-set perplexity. Addressing the issue of syntactic structure, we compare each model’s predictive power against a syntactic generalization score, which is derived from a battery of targeted syntactic evaluation tests following Futrell et al. (2019) and Marvin and Linzen (2018). We find a positive correlation for our eye-tracking dataset, and either no correlation or a negative correlation for our self-paced reading dataset. These results are consistent with the hypothesis that structural bias does not lead to better reading time predictions.
Page permissions have changed
Your browser should refresh shortly…
Renaming wiki...
Wiki page deleted
Press Confirm to return to the project wiki home page.
Connected to the collaborative wiki
This page is currently connected to the collaborative wiki. All edits made will be visible to contributors with write permission in real time. Changes will be stored but not published until you click the "Save" button.
Connecting to the collaborative wiki
This page is currently attempting to connect to the collaborative wiki. You may continue to make edits. Changes will not be saved until you press the "Save" button.
Collaborative wiki is unavailable
The collaborative wiki is currently unavailable. You may continue to make edits. Changes will not be saved until you press the "Save" button.
Browser unsupported
Your browser does not support collaborative editing. You may continue to make edits. Changes will not be saved until you press the "Save" button.
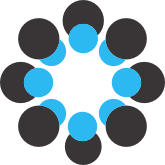
Start managing your projects on the OSF today.
Free and easy to use, the Open Science Framework supports the entire research lifecycle: planning, execution, reporting, archiving, and discovery.
Copyright © 2011-2025
Center for Open Science
|
Terms of Use
|
Privacy Policy
|
Status
|
API
TOP Guidelines
|
Reproducibility Project: Psychology
|
Reproducibility Project: Cancer Biology